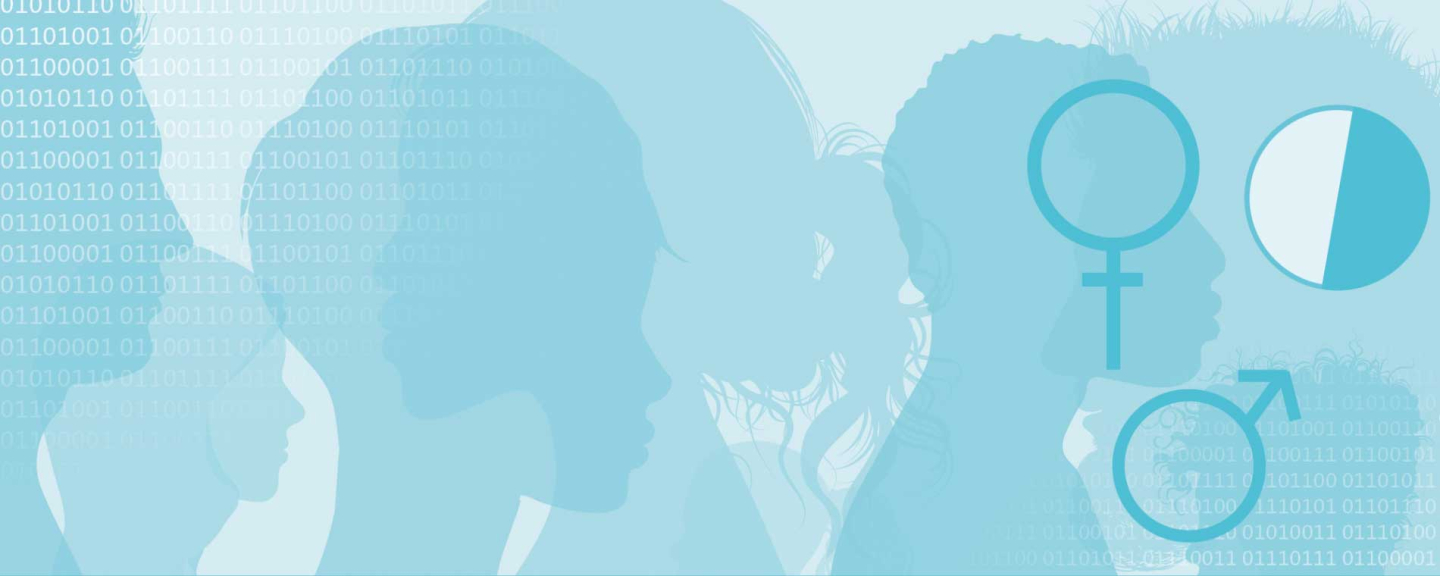
Melita - stock.adobe.com
The hidden bias in AI: Programmed prejudice
Algorithms exhibit biases that may pose a threat to social cohesion. A collaborative research project led by Professor Dietmar Hübner is looking for solutions to a serious problem that will become increasingly pressing in the future.
Job application software, for example, can be a source of discrimination against people of color and women; search engines erroneously deliver discriminatory images. It has long been clear that something must be done about the prejudices hidden in algorithms. Dietmar Hübner, professor of philosophy at the University of Hanover, is doing research on this topic, supported by colleagues in the fields of computer science, law and philosophy who are seeking to develop a common approach to overcoming the problem.
There is no such thing as an objective judgment
Most people concede that their judgments are not always entirely objective: We tend to mix facts, half-truths, prejudices and gut feelings into a general picture on the basis of which we then reach decisions – hoping to be right in the end. No one makes thoroughly objective judgments. To get to grips with this problem, we are increasingly shifting the responsibility to machines in the most diverse areas of life. Computers and algorithms steer decisions that impact on people's creditworthiness, equity investments and even the newspaper articles we read.
The power of algorithms is growing rapidly. Computers are increasingly involved in evaluating people – even on sensitive issues that may be crucial to determining our lives. For example, they judge whether a job application should lead to an interview or whether someone is fit to study. But is it a good idea to leave these decisions to machines?
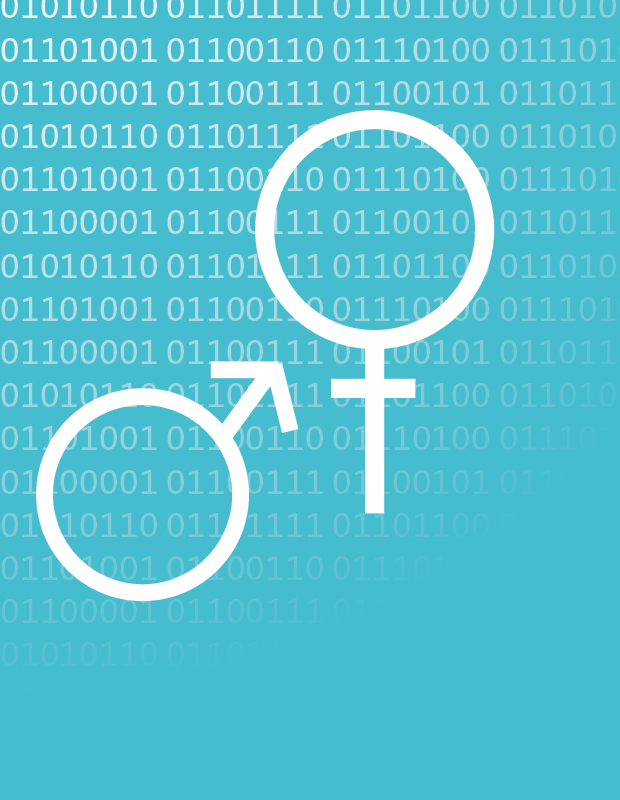
An Amazon software from 2014 that used artificial intelligence to rank applicants for a job was recognized four years later as discriminatory: the algorithm discriminated against female applicants.
Are computing machines really more rational?
After all – at least so we believe – algorithms are neither emotional nor do they harbor subliminal prejudices. It is therefore understandable to think that a machine like the computer will result in important judgments being more objective and thus fairer.
"Unfortunately, this is not always the case. The use of artificial intelligence does not cause prejudices to disappear. Sometimes it even preserves and reinforces them," says Dietmar Hübner, who is studying the phenomenon of machine bias. According to him, decisions made by computers and algorithms can be as racist, misogynistic and discriminatory as those made by humans.
In the interdisciplinary research project "Bias and Discrimination in Big Data and Algorithmic Processing. Philosophical Assessments, Legal Dimensions and Technical Solutions" (BIAS), the researchers want to get to the bottom of the causes and effects of this problem and at the same time develop technical and legal concepts to combat it.
There are many examples of how algorithms frequently discriminate against various population groups, i.e. show machine bias. Back in 2014, the US corporate giant Amazon implemented software that used artificial intelligence to rank female and male job applicants. Four years later, it was discovered that the algorithm discriminated against female applicants. In another case, Google's automatic image-sorting software captioned the photo of an African American woman "gorilla". A New Zealand passport office refused to issue Asians with passports because it thought the submitted photos showed that the applicants had their eyes closed.
An algorithm that discriminates
"A particularly disturbing example is a software called Compas, which supports US judges in sentencing criminal offenders," says Hübner. Compas predicted a higher probability of recidivism for Afro-American offenders than for white persons, measuring the risk in percentage terms. This is clearly misleading – some may of course never again find themselves on the wrong side of the law.
"The results were disconcerting," says Hübner. For example, Compas gave 18-year-old Brisha Borden a score of 8 for a bicycle theft, which corresponds to a very high risk of recidivism. At the same time, a man already convicted for other thefts and more serious crimes received a clement 3 points. The obvious difference between these two people was skin colour – Brisha Borden is black.
Artificial intelligence is like a knife. You can hurt yourself with it, but you can also do very useful things, like cutting vegetables.
But how can it happen that a machine reflects and adopts human prejudices? And how can this be prevented? "Artificial intelligence is like a knife. You can hurt yourself with it, but you can also do very useful things, like cutting vegetables," says Bodo Rosenhahn, a computer scientist and professor at the Institute for Information Processing at Leibniz University Hanover. In the BIAS research project, he and his team are developing the technical concepts to solve the problem. Rosenhahn explains that algorithms learn from selected training data: for example, from information about how people have reached decisions in the past. "But if the selection of this training data is inadequate, algorithms may actually reproduce social problems. As a computer scientist, it is my job to find suitable ways to train models and to set the mathematical conditions that guide algorithmic decisions." In many cases, though, the conditions may contradict each other.
I hope to get some moral guidelines and rules [...] so that algorithms can be programmed more fairly in the future..
Put simply, if a certain type of discrimination is prevented on one side, a new one may arise for another group of people on the other side. If, for example, an algorithm is programmed to apply a stricter measure of judgment to suspected offenders, even innocent people may end up behind bars.
Computer scientists have to curate and program data, i.e., make decisions that have far-reaching social consequences. This is where Rosenhahn appreciates the interdisciplinary collaboration at BIAS: "I hope to get some moral guidelines and rules from the ethicists and legal scholars so that algorithms can be programmed more fairly in the future."
Fairness measures are needed
It is the task of philosophy professor Dietmar Hübner and his team to work out these rules, so-called 'fairness measures', and to weigh them against each other. Yet such trade-offs are quite complex: "Notions and concepts of fairness and morality change over time and depend on the respective context," he says. "Arriving at clear definitions is therefore a lengthy process."
The very different ways of thinking and working in the interdisciplinary research project are potentially colliding contrasts: philosophical dialectics and mathematical unambiguity. Computer scientist Eirini Ntoutsi sees this as a challenge – but also a new perspective. "For me as a computer scientist, working with such a broad and context-dependent concept as fairness is indeed unfamiliar," explains another colleague of Bodo Rosenhahn, a professor at the Institute of Electrical Engineering and Computer Science at Leibniz University Hannover. "But I have already learned a lot from working in the BIAS project. Beforehand, when designing technical solutions, I was not sufficiently aware of the concrete effects my decisions can have on people," she says.
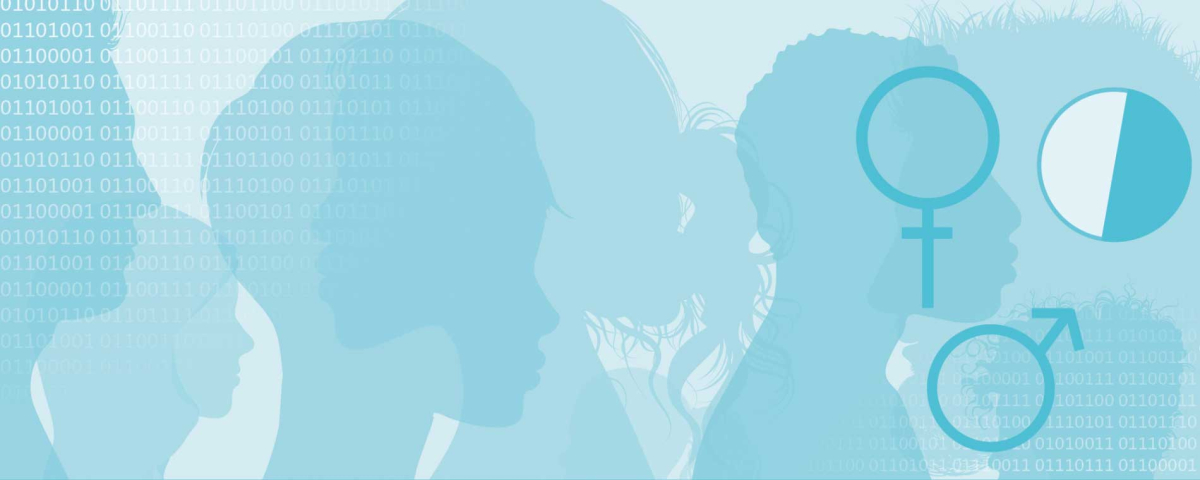
Researchers say that "Faireness measurements" are needed.
Here, trust is misplaced
Blind trust in technology can be problematic: We humans are well aware that we make mistakes and can be misled by emotions. That is why we consult with each other, form diverse committees, appoint equal opportunity officers, rely on majority voting and enact anti-discrimination laws. None of this applies to algorithms – not yet, at least.
Discrimination by algorithms will continue to play a role in Germany, especially in the private and corporate sector.
Legal scholar Christian Heinze wants to change that. Together with his legal team, he forms the third research group involved in BIAS. Heinze is looking at how legal standards and laws could protect people from unequal treatment by algorithms. "Discrimination by algorithms will continue to play a role in Germany, especially in the private and corporate sectors," explains the professor at the Institute for German and European Corporate and Business Law in Heidelberg. Already, about five percent of all German companies make use of artificial intelligence, for example when hiring staff or granting loans. "The Anti-Discrimination Act prohibits discrimination on the basis of ethnic origin or gender, for example," he says. But in the case of algorithms in the private sector, he says, discrimination is often not even apparent because it happens indirectly. For example, he says, if an algorithm is programmed to weed out all applicants for a job who don not wish to work full-time. "Then that algorithm indirectly discriminates against women because women make up the vast majority of part-time workers."
So, what is the best way to deal with this problem in law? Heinze says it is important that legal scholars also publish on such topics and points out: "Courts don't usually deal with matters of computer science". Addressing the issue of machine bias through interdisciplinary collaboration, he says, means that completely different disciplines are also becoming aware of it. "The ideal thing would be to find a way to translate findings from philosophy and computer science into suitable anti-discrimination legislation.
In some cases, there are sound ethical reasons not to use algorithms
What this might look like is still an open question. According to Dietmar Hübner: "In some cases, there are sound ethical reasons not to use algorithms: not only because of the problematic content of computer predictions but also because of the way such results are arrived at or presented. Especially if the processing paths are not transparent, problems can arise in the application of AI."
Possibly, when all is said and done, we as a society will come to the conclusion that in some areas of life it is better to continue to let humans judge humans – errors included.